Super Resolution – The Next Step in High End Imaging Technology
Apr 27, 2020
Single image super-resolution (SISR) is a common and challenging vague problem, which aims to obtain a high-resolution (HR) output from one of its low-resolution (LR) versions. To solve the SISR problem, recently powerful deep learning algorithms have been employed and achieved the state-of-the-art performance.

The end goal is to recover the high resolution image, which one can resample depending on the input images and imaging model. It is very important for the imaging model for the super resolution to be accurate. An incorrect modeling can degrade the image quality. Deep learning can be easily applied to perform the Super resolution rather than using the traditional approach of using images from multiple cameras or video sequence.
Applications of Super Resolution
A high resolution image is of utmost importance in the field of medical imaging for diagnosis of any healthy issues. Other fields requiring the execution of super resolution imaging include surveillance, forensic imaging, and satellite imaging applications. The only issue is that setup for high resolution imaging is a bit expensive, and not feasible for many owing to limitation of sensor, and optical manufacturing technology. There are basically two commonly used Super Resolution frameworks including SRGAN(based on SRResNet) and SRCNN.

What is the ESRGAN?
The Enhanced Super Resolution Generative Adversarial Networks (ESRGAN) is a seminal work capable of generating realistic textures via single image super resolution. The only drawback is that the details often include unnecessary artifacts. The ESRGAN provides better visual quality with more realistic and natural texture as compared to the traditional SRGAN.
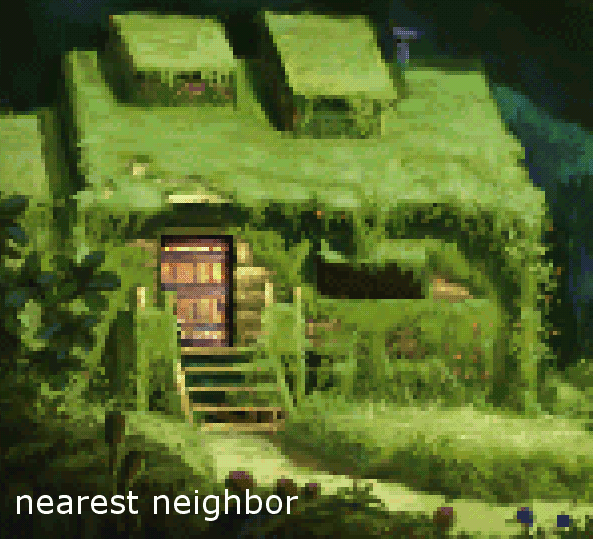
Apart from the breakthroughs in accuracy and speed of single image super-resolution using faster and deeper CNNs (Convolutional Neural Network), the main pending issue is how would one recover the finer texture details when super-resolving at large upscale factors. We present to you the GAN (Generative Adversarial Networks), the framework capable of inferring photo-realistic natural images for a 4x upscaling factor.

There is a discriminator network trained to differ between the super-resolved images and original photo-realistic images. The original authors also utilized a content loss motivated by perceptual similarity instead of the similarity in pixel space. The deep residual network recovers photo-realistic texture from the heavily down-sampled images.

Super Resolution CNN?
The Super Resolution Convolutional Neural Network, also known as the SRCNN is a deep-learning based super resolution method for enhancing image resolution in CT images. The success of this model has been proven when 89 chest CT cases were divided among 45 training cases and 44 external test cases.

Using the SRCNN, a high resolution image was constructed from a low resolution image, which was down-sampled from an original test image. The two image quality metrics were compared with the conventional linear interpolation methods. The image restoration quality of the SRCNN scheme was higher as compared to that of the liner interpolation method.

The high resolution image reconstructed using the SRCNN was restored and comparable to the original image used for reference for a x2 magnification. It indicates that the SRCNN scheme significantly outperformed the linear interpolation methods for improving the image resolution in CT scan images. In short, SRCNN has the potential to create high resolution CT images from standard CT images You can know more about this via the image super resolution technology including:

SRCNN Vs SRGAN – A Simple Comparison
SRCNN is a deep learning network which consists of three parts namely patch extraction and representation, non-linear mapping, and reconstruction. On the other hand, the SRGAN (Super Resolution Generative Adversarial Network) is a seminal work capable of generating realistic textures during single image super resolution. The details are often followed by unpleasant artifacts.
It important to note that SRGAN can be quite creative in its upscaling that it would be easy to get fooled as to believing the output as ground truth. One should be careful when using this tech for security purposes as it can be used to apprehend a look-alike innocent person as opposed to the perpetrator.

Summary
The Super Resolution framework reconstructs a higher resolution image or a sequence from an observed low resolution images. SR has been under development from last three decades for both multi and single frame applications in daily life. Thus, Super Resolution is surely the next step in the evolution of high resolution imaging services in the medical and other sectors. However, it needs to grow to become more accurate, efficient as well as deployable for real-time applications.
To Learn More AI – Check this Link.
Ritesh Kanjee
Augmented Startups
Stay connected with news and updates!
Join our mailing list to receive the latest news and updates from our team.
Don't worry, your information will not be shared.
We hate SPAM. We will never sell your information, for any reason.